Thinking about Chat GPT as a search tool

Like almost everybody in the academic world, I suspect, I’ve been thinking a lot about Chat GPT.
On the one hand, I think the belief that It Will Change Everything is vastly overblown. Instead of a replacement for human professionals, or students, or the end of the essay as a form of communication and evaluation, I think we have to see ChatGPT as the writing equivalent of a calculator — something that provides quick answers to sub-processes in the thinking and research cycle, but that handles only the bits that can be automated. When cheap pocket calculators first came out, I remember people saying that it would destroy math class. But instead, math class changed to incorporate them: because addition, subtraction, multiplication, and even more complicated calculations are not the sum of mathematics.
The same is true of what ChaptGPT does, which is provide integrative answers to queries. Until now, this has been a human task: search engines (and before that, [card] catalogues and indices) could provide links to sources, but, on the whole, they couldn’t integrate the information they contained. What ChatGPT does is integrate information from the web so that you get an answer, rather than a list of resources, to your queries.
The trouble, of course, is that the answer may-or-may-not be true or accurate (though in my queries it has been, with a couple of astounding exceptions, more-or-less acceptable); but also that the answer it provides is very anodyne: ChatGPT is not the place to turn to if you want an insightful answer to a question, or a wise one. It is the place to turn if you want to know what “they” think — i.e. the consensus of the internet.
The result is that ChatGPT answers are generally very boring. They are basic summaries of what people think about a topic, written in unobjectionable language. Sure you can get it to write in limerick format. But if you want it to produce a paragraph on something, do not expect it to win any writing prizes.
My own feeling is that for this reason ChatBots are nowhere near replacing people. If somebody has a job where they are required to produce pages of anodyne prose recounting consensus positions without necessarily knowing if what they are writing is accurate, then I suspect they probably are not a very important cog in the machine anyway and they are better off being replaced by a bot — in much the same way that a lorem ipsum generator like lipsum.com has replaced the need to make up filler text for typesetters by hand.
Likewise, for students, ChatGPT has probably eliminated the F essay in much the same way that calculators have made it hard to fail basic arithmetic assignments: unless it is hallucinating, ChatGPT should be able to produce a not-wrong, grammatical essay on pretty much any topic. But nothing I’ve seen says it should be earning Bs and As (well, maybe except at Yale).
The reason for this all is because ChatGPT is literally a bullshit engine. That is to say, like bullshit, it is supposed to sound good, without having “any particular concern for truth, clarity, or meaning.” The Large Language Model works by predicting what the mostly likely next word should be in a sentence according to its training set. Since its training set is real world documents produced by people who by-and-large try to be accurate, this means that this is also often a way of averaging meaning. But since the goal is to “sound like” what it finds in its training set, it is only accidental that this is normally true. ChatGPT answers are the consensus of what it’s model says an answer to a specific question might be; and that, coincidentally, is also likely to mean the content will be similar too.
So when we use ChaptGPT to produce text for us, we are actually trying to take advantage of a by-product of its model. The bot is not designed to produce correct answers, but to sound like it does. But a lot of the time (presumably) sounding like you produce correct answers is the same as producing correct answers. And sometimes it isn’t. If you decide you want the bot to produce your essays, then you are running a risk that this disconnect between how the bot works and what you are supposed to do will result in something that produces a mess. And even if it doesn’t produce a mess, it almost certainly won’t produce something original-sounding, since sounding original is the opposite of what it is supposed to be doing.
Thinking further along this line, then, there are some things you can do with it that take advantage of what the bot is supposed to be doing — that is to say, take advantage of the fact that it is not supposed to sound original.
One of these is to use it as a way of integrating search results. I found a great example of this the other day when I was researching literary agents. When you write a query letter to a literary agent, one of the goals is to fit your proposal into their catalogue of authors — show how you are like, but not the same as, the other authors they represent.
Doing this is a tedious task: most agents either don’t list all the authors they have represented or, if they do, simply list their names with, at most and if you are lucky, a link to the author in question’s home page. And most authors, it seems are very bad at running their own web-pages, meaning they are mostly broken or missing.
The result is that, as a human, you are faced with a massive research task if you want to do a good job of filling in the authors you are most like:
- Go the agents site and find their list of author names
- Go to the few author sites that are working and see what kind of work they have produced (most of which will not be like yours)
- Go to a search engine and look up the titles that sound like they might be like yours and hope you can find a synopsis.
- Repeat #1 with the next author on the list
- Repeat # 4 with the next agent on your list.
Yesterday, when I was researching authors, I realised that this is the perfect work for a bot: it requires, in essence, matching words with each other: agent-to-author, author-to-books, and books-to-genre.
And indeed it worked reasonably well. I had a list of agents sorted by genre they represent from QueryTracker (though I realise now that I could probably have compiled this list using ChatGPT itself). For each author, I then asked “Historically which thriller writers has agent x represented” (I had to phrase it this way, because ChatGPT took the question “which thriller writers does agent x represent” as a request for proprietary data). The result was that I would get a list of the thriller writers most commonly associated with each agent, as well as in most cases, a list of their most commonly referenced book titles. I didn’t have to wade through writers of other genres, or even try to figure out genres. And then if I wanted more information on a likely comparator for my own work, I ask ChatGPT to tell me about that author’s work: “tell me more about thrillers written by Tana French” — which would then produce a synopsis of their top five-to-ten novels or series.
Another approach I took was to scrape the lists of clients from each house and then ask ChatGPT “Which of these novelists write thrillers?” Here, initially on ChatGPT 3.0, I probably got about 50% hallucination, but at least some real names (as checked against Google); on ChatGPT 4.0, it seems to be more accurate.
Another thing that really exploits ChatGPTs training model is using it to assess how public relations campaigns are going/have gone. Once again, this is because ChatGPT’s “answers” are really just the relationships between words it finds out there most commonly on the internet.
I realised this the other day when I asked ChatGPT about the job action last year at the “University of Lethbridge“https://www.ulfa.ca/taking-action/job-action-updates/:
What were the issues of the University of Lethbridge strike
The University of Lethbridge Faculty Association (ULFA) went on strike in January 2022 due to a dispute over a new collective bargaining agreement with the university’s administration. Some of the key issues in the negotiations included salary increases, workload protections, job security for sessional lecturers, and protection of academic freedom. The ULFA was seeking fair compensation for its members, as well as more support for its sessional lecturers who are employed on a contractual basis. The university administration argued that the proposed salary increases were not sustainable and that it needed to prioritize financial stability. Ultimately, both sides made concessions during the negotiations, leading to a tentative agreement and the end of the strike.
This is interesting, because the Bot’s summary of the strike is very much in-line with ULFA’s position, rather than the Board of Governors’. According to the Bot, the issues were salary increases, workload protection, job security, and protection of academic freedom, not management rights (which the Board emphasised in their communications). Even in the summary of the two positions, the positions are about the issues raised by the union rather than management: “The ULFA was seeking fair compensation for its members, as well as more support for its sessional lecturers who are employed on a contractual basis. The university administration argued that the proposed salary increases were not sustainable and that it needed to prioritize financial stability.”
What this suggests is a new battleground in public relations: Integration Engine Optimization, or attempting to ensure that your version of events is seen as the most likely by the large language model algorithm. In this case, I suspect we “won” the Bot’s answer because we did a much better job getting our message out across various channels: while the Board side restricted itself largely to its own blog and the occasional press-release, we were much more active in the media space: we had our own blogs and press-releases, but also did many more interviews across different news media, and did a better job of getting allies to reflect our positions in their own posts and social media — all of which presumably increased the weighting of “our” combination of words rather than the Board’s, since “our” words were combined across a larger number of websites and posts than “theirs” were.
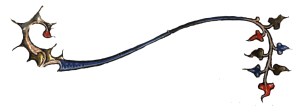